What is Responsible AI? Principles, Practices, and Examples
Artificial Intelligence (AI) has transformed various industries, including healthcare, finance, and customer service, enhancing efficiency and decision-making. However, as AI technology advances, concerns about fairness, privacy, transparency, and accountability have become more pressing. Biased algorithms, safety risks, and opaque decision-making processes highlight the need for ethical considerations in AI development and deployment.
This research will explore key concepts of responsible AI, provide real-world examples, and outline practical steps for integrating ethical frameworks into AI development at both individual and organizational levels.
What is Responsible AI? Definition and Importance
Responsible AI refers to use, adoption, and design of artificial intelligence systems anchored in societal and ethical values, equity, and firm commitment to being accountable. Responsible practices promote and augment human decision-making without replacing it, reducing the likelihood of bias, discrimination, and data breaches.
Responsible artificial intelligence not only means developing complex AI systems but also imbuing values into every step of the lifecycle of AI—from data collection and model training up to usage and end-user experience. By prioritizing fairness, explainability, and accountability, we can ensure trust in such AI systems and, eventually, positive impacts on society.
The growing use of artificial intelligence has also been accompanied by a rise in ethical questions. Artificial intelligence systems too often rely on large datasets, and biased datasets can generate unequal and harmful consequences. A biased AI tool, for example, for employment can disadvantage some demographic categories, and untransparent AI processes in industries such as healthcare and finance can result in reduced accountability and trust.
By implementing responsible AI practices, organizations can:
- Mitigate bias in artificial intelligence systems by ensuring training data remains diverse and representative.
- Enhance transparency with the clarification and understanding of artificial intelligence-driven decisions.
- Strengthen accountability with the institution of defined protocols for developing and implementing artificial intelligence.
- Enhance confidence among stakeholders and end-users by assuring that artificial intelligence complies with ethical values and legal frameworks.
- Promote fairness by preventing discrimination and ensuring AI benefits all communities.
Real-World Examples of Responsible AI
Responsible AI is already being applied across various industries:
- Healthcare: AI-powered clinical decision-support devices that help physicians review clinical data while ensuring both confidentiality and visibility.
- Financial fraud prevention systems: such as those created by FICO, assess real-time behavior of transactions for greater security with minimal disruption of optimal user experience.
- Education: AI tutors personalize learning experiences while maintaining transparency in their recommendations.
While predictive policing systems seek to improve resource allocation and reduce bias, studies indicate that biased historical data can lead to disproportionate targeting of certain communities.
- Environment: Artificial intelligence-powered climatic projections predict changes and inform the crafting of sustainability plans.
- Transportation: While Waymo and other autonomous vehicle companies implement responsible AI systems to enhance safety and reliability, concerns remain regarding real-world performance, regulatory frameworks, and accident risks.
By embracing responsible and accountable practices of artificial intelligence, businesses can ensure their artificial intelligence systems not only for their purposes but for the collective advantage of society. With continuing advancements in artificial intelligence, being accountable becomes a key approach for gaining trust and realizing sustained performance.
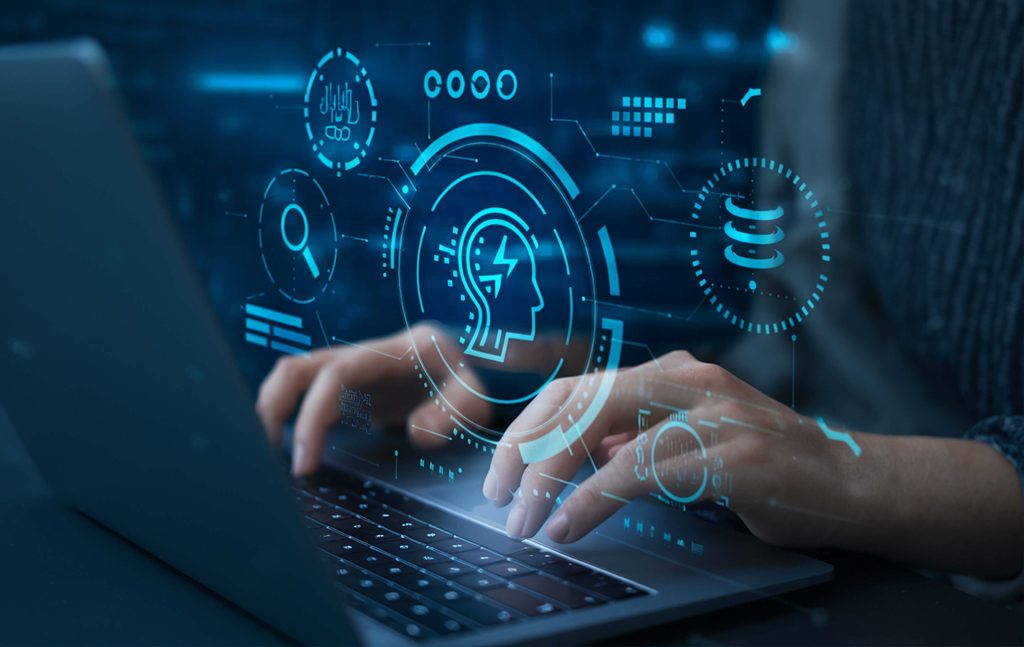
Key Principles of Responsible AI Practices
As artificial intelligence (AI) becomes more influential across different industries and on decision-making, there needs to be responsible practices of AI. Effective encryption techniques should protect data when in storage and when being transmitted. Regular security audits should identify vulnerabilities and ensure compliance with privacy laws such as GDPR.
AI security also includes protections against cyberattacks and adversarial manipulations that could compromise the integrity of AI models.
1. Equity and Preconceived Ideas
Artificial Intelligence has the mandate of guaranteeing equity for everyone, regardless of race, gender, and socioeconomic status. However, such prejudices can find their way into artificial intelligence systems inadvertently via biased training data and algorithmic code defects.
To foster equity:
- Artificial intelligence systems should be created with data being extensive and reflective.
- Bias-aware algorithms must be developed to reduce disparities in AI-driven decisions.
- Regular assessments should be conducted for recognizing and resolving prejudices inherent in artificial intelligence systems.
- By employing equity, artificial intelligence can be best utilised in key areas of employment, finances, and healthcare.
2. Lucidity and Openness
AI systems need to be transparent in their decision-making processes, enabling trust and enabling people to understand why they made their decision. The demand for explainability becomes more urgent when dealing with critical use cases, such as banking, medicine, and legal practice. Explainable AI (XAI) methods are what make human-understandable decisions of AI.
3. Responsibility and Accountability
With AI playing a growing role in decision-making, ensuring accountability is crucial. Why are responsible AI practices important to an organization, and why should individuals developing AI systems be responsible for their outcomes?
This requires:
- Establishing clear ownership means assigning responsibility between AI builders, companies, and governing bodies.
- Audit trails to track AI decisions, making it possible to investigate errors or biases.
- Feedback mechanisms allow people to challenge artificial intelligence-driven decisions and request more detail.
- Ethical AI review panels can also ensure artificial intelligence complies with legal and ethical standards.
4. Privacy and Security
Artificial Intelligence systems regularly process private personal data, and hence, privacy and security must be key factors in ethical use. To preclude illicit access and data misuse:
The principle of data minimization requires collecting only necessary data.
Effective encryption methods need not only protect data when stored but also when transmitted.
Regular security audits should discover such vulnerabilities and guarantee data-privacy laws, such as the GDPR, are being obeyed. Also, artificial intelligence security deals with protections against adversarial manipulations and cybersecurity breaches, both of which compromise the trust vested in the AI model.
5. Stability and Reliability
Artificial intelligence systems need to be able to perform under different scenarios, including unexpected ones.
Ensuring reliability means:
- Performing stress tests on artificial intelligence systems for testing their responses to unexpected events and abnormalities.
- The ability to resist adversarial attacks, hence protecting oneself against being manipulated.
- Continuous monitoring and updates to keep AI systems safe and effective over time.
- Dependable artificial intelligence systems foster trust and ensure artificial intelligence solutions maintain their safety and reliability in real-world environments.
Responsible AI Frameworks and Their Applications
As artificial intelligence becomes more integral to corporate practices, its use becomes necessary. A strategically grounded approach towards artificial intelligence offers a holistic view of its growth, with equity, transparency, accountability, and compliance being intrinsic goals. Its use can be beneficial for helping companies comply with regulations, manage risk, and gain trust with solutions created with artificial intelligence.
While AI ethics provides guiding values, responsible artificial intelligence frameworks outline the steps for implementing such values. Responsible frameworks for artificial intelligence guarantee that ethical values inform every step of an artificial intelligence system’s lifecycle, from its conception and development to its use and ongoing review.
The approach enables companies to address possible problems such as bias, data confidentiality, and legal requirements ahead of their becoming major issues.
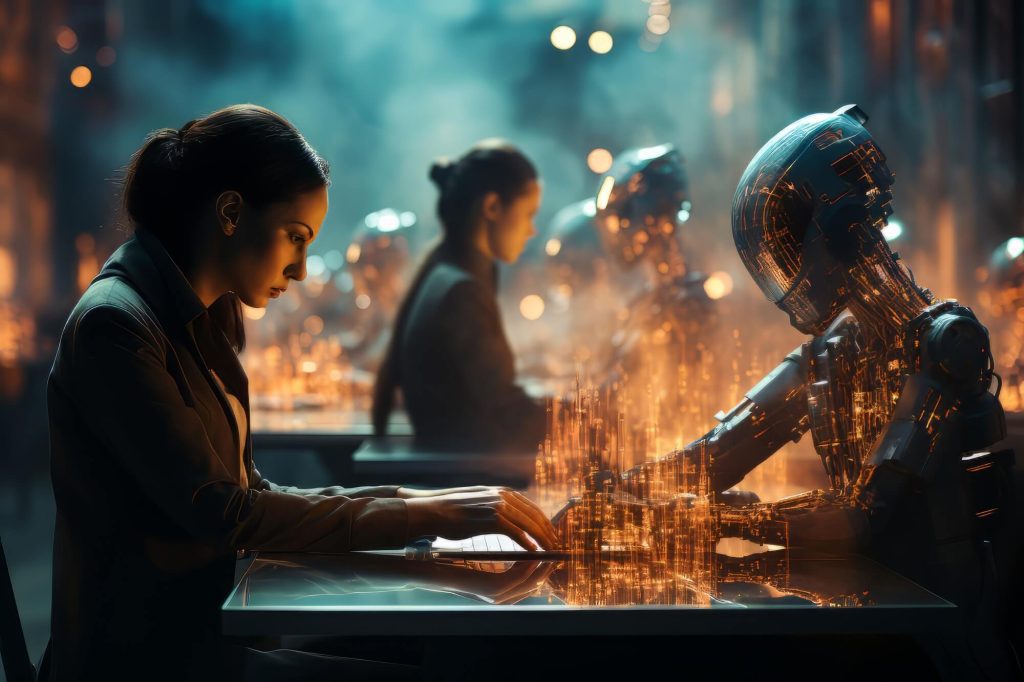
Key Components of a Responsible AI Framework
- Governance and Oversight: Creating governance for artificial intelligence ensures proper governance and accountability. A governance group, including ethicists, data experts, corporate leaders, and legal specialists, guides the development of AI and ensures compliance with laws like GDPR and HIPAA
Application: In the context of responsible AI in the enterprise, and more so for financial institutions, there exists a huge drive for the use of governance frameworks for artificial intelligence. The frameworks are critical for auditing the systems of automatic decision-making, prevalent in the industry, and ensuring fair practices of lending and prevention of biased scoring of credits.
- Ethical Risk Measurement: It is critical for AI systems to be thoroughly and scrupulously analyzed for identifying possible risks that can be created, with major concerns being related to fairness, privacy, and the proliferation of disinformation.
Application: While AI-driven job portals can leverage responsible AI solutions like IBM’s AI Fairness 360 to address hiring biases, there is limited evidence of their consistent and verifiable implementation.
Using such revolutionary technologies, such portals attempt to guarantee equal opportunities for every job searcher, irrespective of their personal background, traits, and individual traits.
- Transparency and Interpretability: It is of utmost importance that artificial intelligence systems’ decision-making be not only understandable but also fully explainable.
- Organizations owe a duty of care to ensure their artificial intelligence models can explain the reason for their decision-making processes very clearly for different stakeholders, including the regulators who manage their conformity, and end-users who depend on these systems for knowledge-driven choices.
- In the customer service industry, artificial intelligence chatbots use extensive decision logs in order to explain their responses. The practice not only ensures transparency but also contributes towards helping increase customer trust in such computer systems.
- Continuous Monitoring and Improvement: AI systems should be continuously monitored to detect biases, inefficiencies, and emerging risks. Regular audits, retraining, and updates help maintain ethical AI practices.
Application: Speech recognition AI in virtual assistants undergoes periodic testing to ensure accurate voice recognition across different accents, minimizing discrimination in automated services.
Strategic Integration of AI Frameworks
A responsible artificial intelligence infrastructure extends considerably beyond ethical matters; it means incorporating governance of AI into the overall corporate strategy. This needs to be done in order to ensure conformity with applicable regulations, promote process transparency, and drive long-term sustainability for the overall firm.
- Aligning Artificial Intelligence with Business Goals – It becomes necessary for AI not only to augment decision-making processes but also to strengthen the abilities of automation, maintaining strict conformity with ethical factors and regulatory requirements. With regard to responsible adoption of AI in the corporate environment, banks and other financial institutions are more and more relying on AI-based risk analysis techniques for streamlining their investment plans. Apart from maximizing returns, this process also ensures AML compliance requirements, hence maintaining the purity of financial deals.
- Managing AI Expectations – AI is not infallible; it requires high-quality data and human oversight. Responsible AI solutions in retail continuously refine recommendation models to avoid bias and irrelevance.
- Ensuring Algorithmic Transparency – Organizations must be very diligent in documenting their artificial intelligence decision-making processes to maintain trust and accountability between their users and stakeholders. With regard to banking, and more specifically with systems of artificial intelligence-driven credit scoring, there needs to be provision of explicit reasons for approval and rejection of credits for purposes of staying compliant with anti-discrimination laws protecting consumers.
- Regulatory Compliance & Ethical Adoption – AI governance must adapt to evolving regulations like GDPR and HIPAA. AI-driven customer support must safeguard user data while ensuring efficiency.
By effectively combining governance frameworks, end-to-end monitoring systems, and stringent ethical protections, companies can scale up AI innovation responsibly. This ensures critical values of fairness, accountability, and compliance are sustained from the very beginning.
Examples of Responsible Artificial Intelligence in Action
Artificial Intelligence (AI) is now firmly integrated into various sectors, enhancing both innovation and efficiency as well as aligning societal values and ethical issues with increasing focus on responsible AI application.
The examples cited herein demonstrate the use of responsible AI in different industries, where techniques of AI are being optimized for developing solutions with a responsibility-driven approach.
1. Healthcare: Improving Diagnosis and Enriching Patient Experiences
In medicine, reliable AI systems analyze vast amounts of data to enhance early disease detection and improve patient outcomes. Besides, AI personalizes treatment protocols and monitors patients’ vital signs with the potential to save billions in healthcare expenses annually. These AI methods include machine learning algorithms that sort through large data sets to identify patterns and predict health outcomes, with the hope that AI solutions are effective and ethical.
2. Finance: Fraud Detection and Risk Management
The financial industry taps into the accountable power of AI, uncovering fraudulent behavior with a precise examination of trends in transactions, protecting the institution and its clients. Beyond this, AI plays a key role in risk and compliance, skillfully filtering large data sets for signs of threats and reducing the costs of compliance.
Anomaly detection and predictive data mining techniques are utilized to find outlier trends and ward off potential monetary dangers, assuring not only the efficacy of the solutions but also their conformity with ethical practices.
3. Education: Personalized Learning and Administrative Efficiency
Educational institutions use the abilities of AI to design individualized teaching experiences with the use of student performance data, maintaining confidentiality and guaranteeing transparency. Administrative tasks such as student attendance and resource utilization are also optimized with the use of AI, releasing significant time for teachers to dedicate to teaching.
Natural language and data mining techniques are utilized for tailoring teaching material to the individual needs and learning style of every learner and protecting the effectiveness and morality of solutions offered.
4. Customer Service: Call Centers Transmuted
Responsible AI revolutionizes customer service for call centers, ushering in a future of smart, traceable, and personalized experiences. The performance is also monitored with utmost care, generating useful feedback for personalized coaching and unbiased assessment processes.
Methods including voice and sentiment analysis are utilized for measuring customer sentiments, enabling responses to be accordingly matched, and hence solutions being effective and ethical.
5. Retail: Inventory Management and Customer Experience
In the retail industry, artificial intelligence elegantly simplifies stock management, with data-driven insights into customer buying habits, waste minimized, and satisfaction maximized.
In addition, recommendation systems designed with artificial intelligence power enhance shopping experiences with personalized recommendations, with the backdrop of responsible AI. Using predictive statistics and collaborative filtering methods, retailers can not only use artificial intelligence solutions for their predictive and personalized recommendations, but also with ethics.
These examples showcase the ways industries are accepting responsible artificial intelligence solutions, implementing different methods of performance improvement with a firm commitment towards societal values and ethical practices.
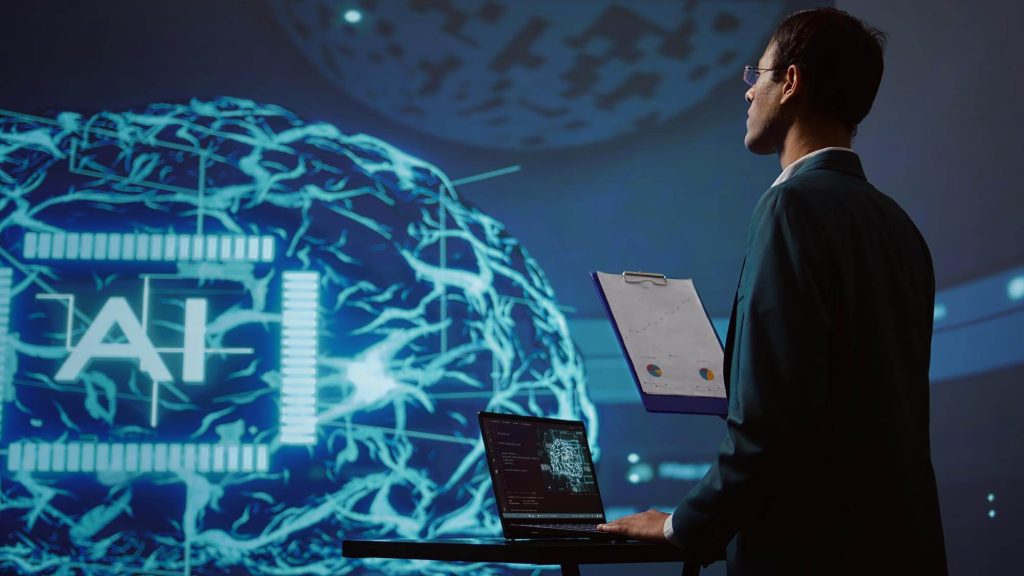
Challenges in Implementing Responsible AI Solutions
The adoption of ethical artificial intelligence ensures such systems perform both morally and effectively in many industries. However, there are a variety of barriers that limit the smooth adoption of responsible solutions for artificial intelligence. Following are five of the main factors, and possible solutions for their removal.
1. Technical Complexity
Creating AI systems that are both capable and ethical requires a very high technical competence. The process of identifying and resolving bias in AI systems is complex, involving specific skill in machine learning and also ethics.
Strategy: Invest in multidisciplinary squads of data scientists, ethicists, and domain specialists. The strategy ensures rigorous testing for bias and also ensures conformity with ethical requirements.
2. Absence of Regulations
The absence of international and national artificial intelligence standards creates a perception of insecurity, and therefore, regulations for accountable practices for artificial intelligence are not easy to implement.
To be proactively engaged with industry associations and standardization institutes and assisting in influencing regulations on AI, is critical. The setting of international standards, including ISO/IEC 42001, can offer a solid framework for addressing both the dangers and opportunities of AI.
3. Conflicts of Interest
Organizations might also prioritize profits more than ethics, and this can create conflicts between responsibility and innovations when developing artificial intelligence.
Strategy: From the earliest point, infuse ethical factors into the very fabric of the innovation process. Establish in-house ethics panels charged with overseeing artificial intelligence projects, ensuring that ethical frameworks harmonize with business goals.
4. Digital Inclusion
A significant challenge remains with regard to guaranteeing access for every individual, regardless of their socioeconomic status, to the advantages of AI.
Strategy: From the beginning, design AI solutions with inclusivity. This means understanding and meeting the varying needs of people from the beginning and working assiduously towards the elimination of prejudices and discrimination, ending exclusions.
5. Environmental Impact
Training AI models is often energy-intensive, raising concerns about environmental sustainability.
Strategy: Invest in research and developing more effective AI devices and algorithms. Apart from this, using alternate renewable energy sources for data centers can further significantly minimize the ecological footprint of these systems.
Handling such challenges demands united action from all the stakeholders involved in the production and application of artificial intelligence. However emphasizing careful practices can enable organizations to employ the full strength of this dynamic tool without jeopardizing ethical and social values.
FAQs
What is Responsible AI, and why is it important?
Responsible AI is ethics-centric, simplicity-first AI design and usage with close focus on humanity and social value compatibility. Having responsible implementation of AI is most crucial to answer concerns about bias, discrimination, and privacy infringement build confidence, and enable people’s acceptance of AI technology. Becoming responsible doesn’t simply minimize harm but also maintains innovation for the better at a societal level.
Who is responsible for implementing Responsible AI practices?
Making sure responsible AI is more than the work of a single group—it’s a collective effort. From AI creators and deployers to policymakers and regulators, everyone has a stake. It requires a group effort to design and implement ethical standards that allow AI to do good for society while reducing the risk of potential harm. When all parties are working together, AI can be a force for good instead of a cause of unintended harm.
What is something Responsible AI can help mitigate?
Responsible AI works towards reducing the security threats associated with solutions involving AI. Harmonizing stringent security practices with ethics reduces the vulnerability that may be exploited for ill purposes, hence protecting the end-user and the integrity of their data.
What responsibilities are associated with Artificial Intelligence?
Artificial Intelligence carries responsibilities including ensuring transparency, accountability, and fairness in its applications. Developers and organizations must ensure that AI systems do not perpetuate biases, respect user privacy, and operate securely to prevent misuse or harm.
What can Responsible AI mitigate?
Responsible AI can reduce unwanted biases in AI systems so that fair and unbiased outcomes are produced in a wide range of applications. Through the establishment of ethical guidelines and ongoing oversight, Responsible AI deals with problems such as discrimination and inequality generated by biased data or algorithms.